Category: Blog
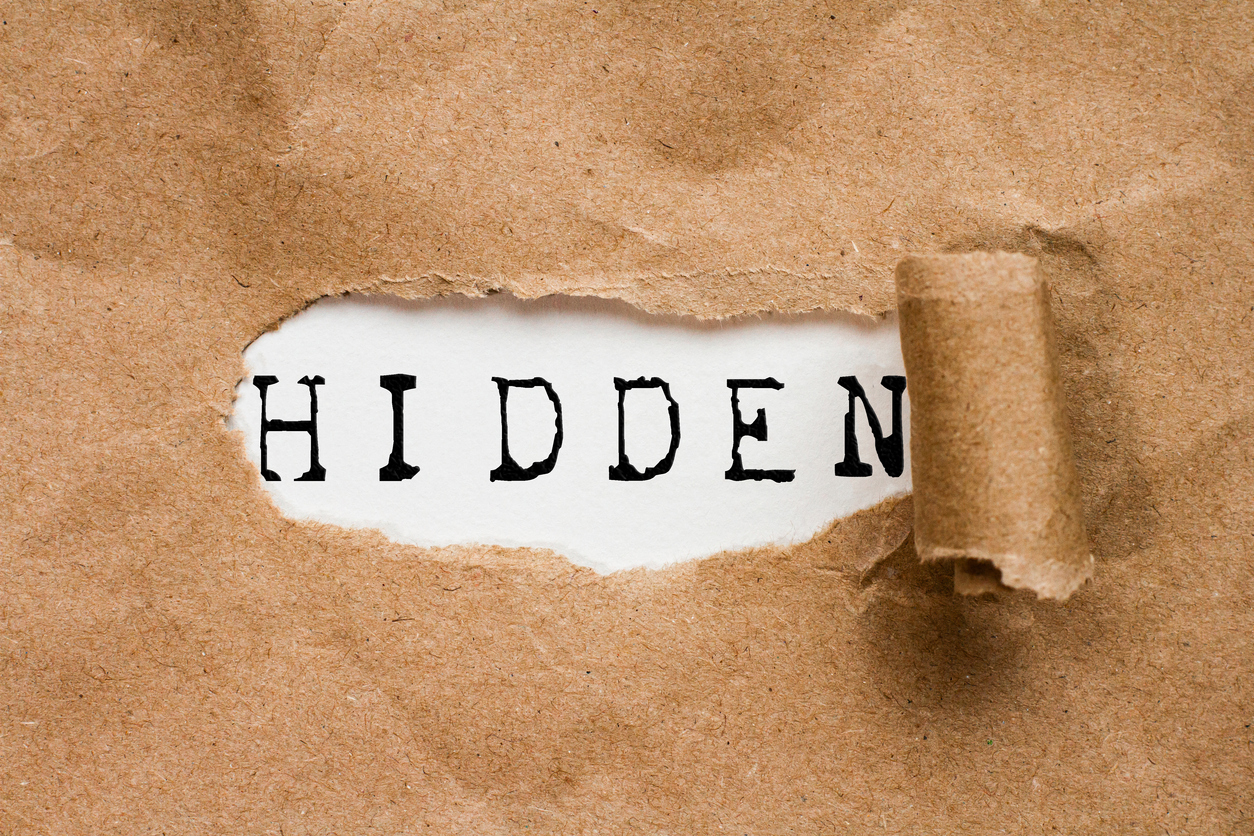
Speed, accuracy, and efficiency are non-negotiables when it comes to software development. Yet, many organizations still rely on manual testing, unaware of the hidden costs that weigh down their QA efforts. From excessive time spent on repetitive tasks to the high cost of maintaining outdated test scripts, manual testing creates bottlenecks that slow down releases
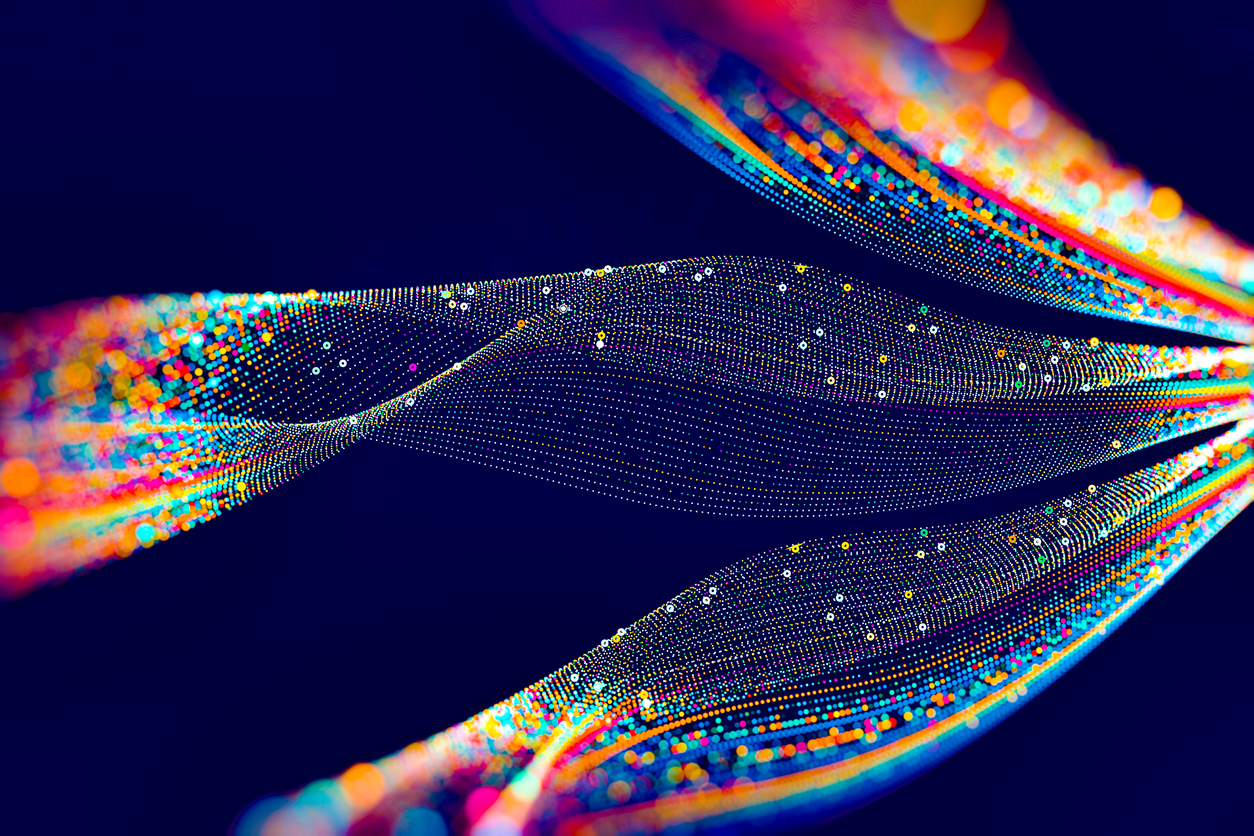
The landscape of software QA is undergoing a seismic shift. Traditional test automation, once considered the gold standard, is quickly becoming outdated as AI-first automation redefines the industry. Organizations that fail to adopt AI-driven QA risk falling behind in speed, efficiency, and overall software quality. The Limitations of Traditional Automation For decades, QA teams have
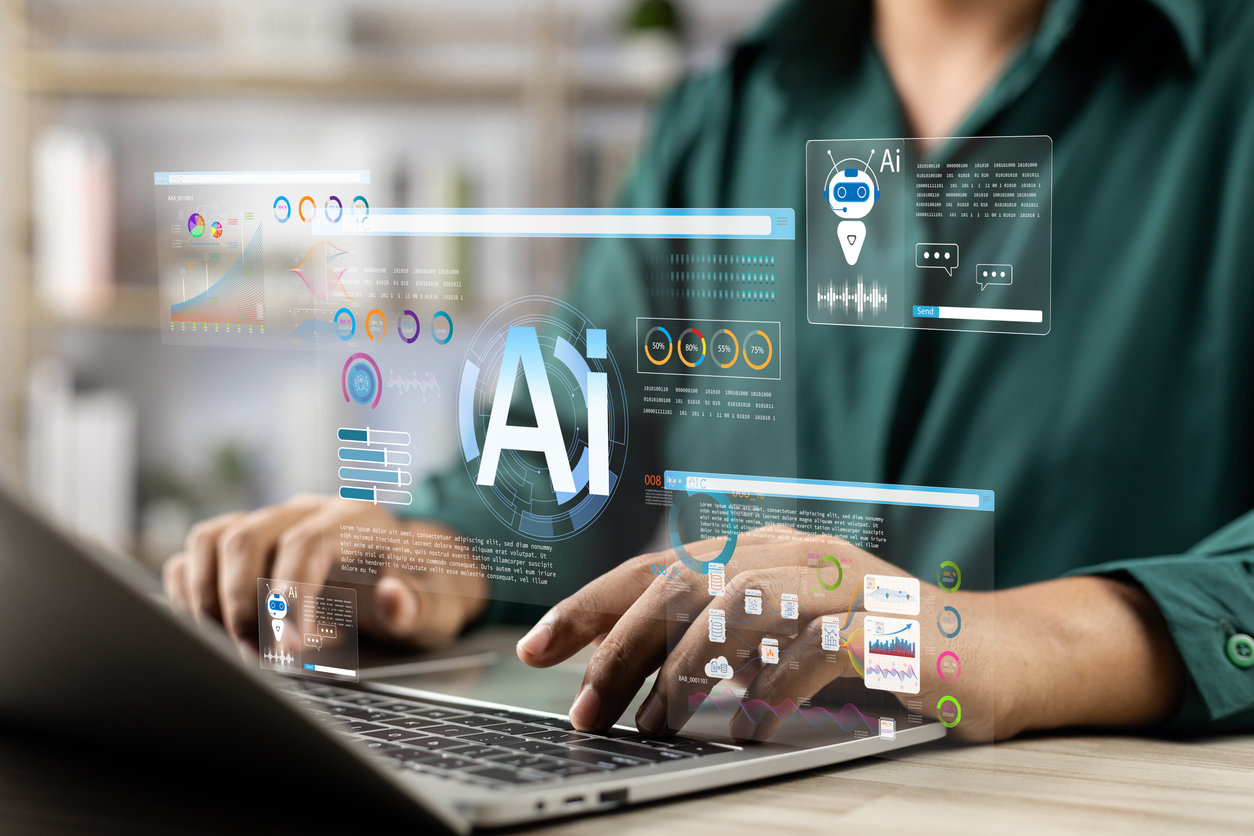
The rapid evolution of software development has placed immense pressure on QA teams to deliver faster, more efficient, and cost-effective testing solutions. Traditional testing methods, reliant on manual testers and script-based automation, struggle to keep pace with the speed of modern software releases. Enter AI-first test automation, a game-changing innovation that is transforming the way organizations
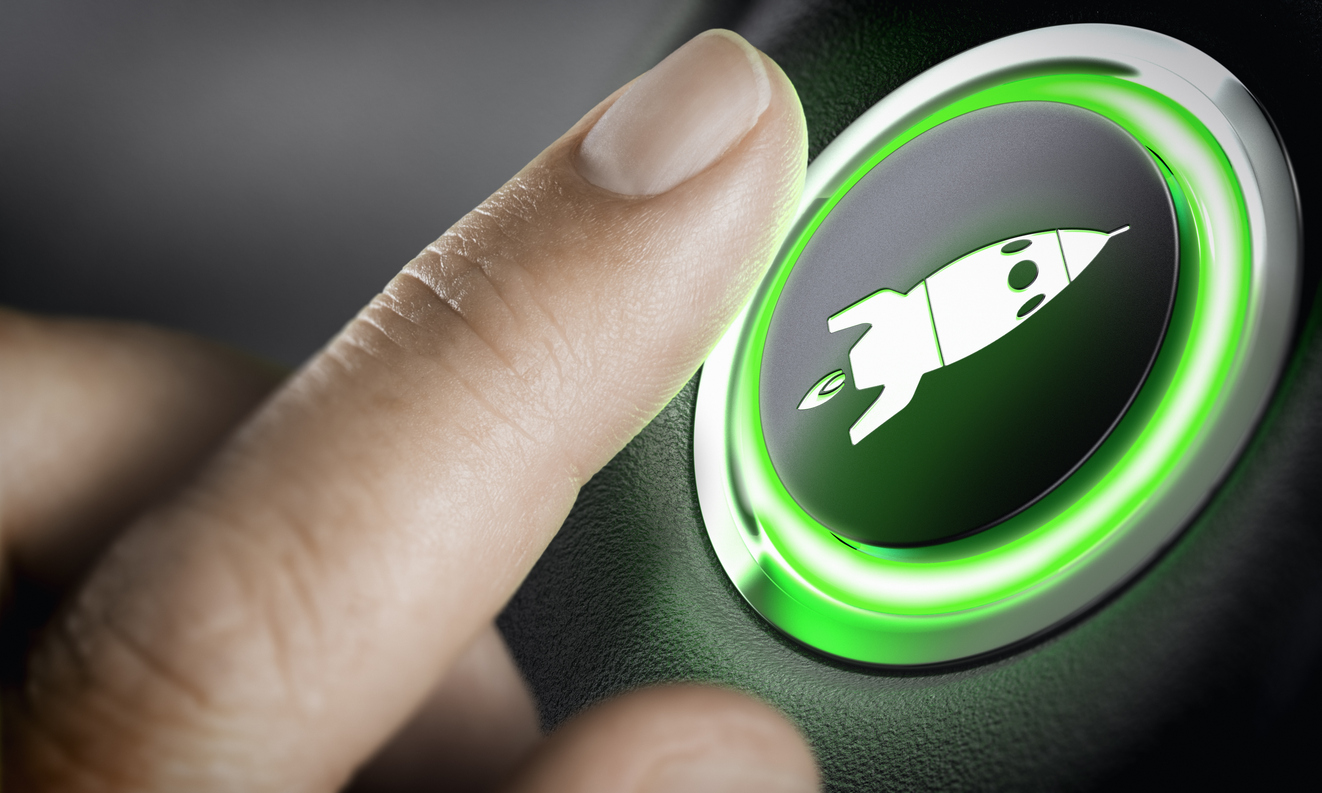
Organizations face the dual challenge of ensuring comprehensive test coverage while accelerating time-to-market. Traditional software testing methods, relying heavily on manual effort and human-led scripting, often struggle to keep up with rapid release cycles. Enter Appvance IQ (AIQ)— an first AI First test automation platform that revolutionizes quality assurance by maximizing test coverage and minimizing testing
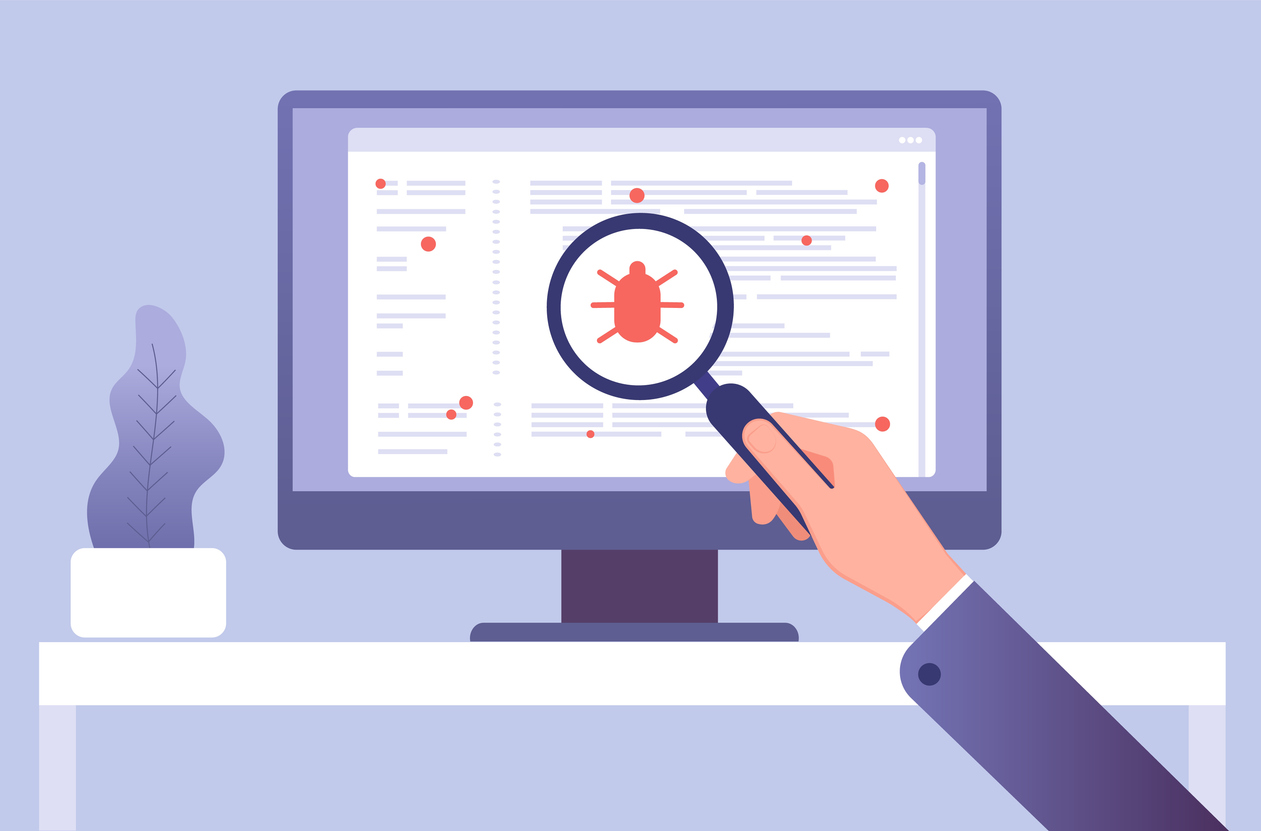
Software is the backbone of businesses across industries in the digital economy. Whether it’s a mobile banking app, an e-commerce platform, or an enterprise resource management system, the expectation for seamless, defect-free software has never been higher. A single bug in your software can not only disrupt user experiences but also tarnish your brand reputation
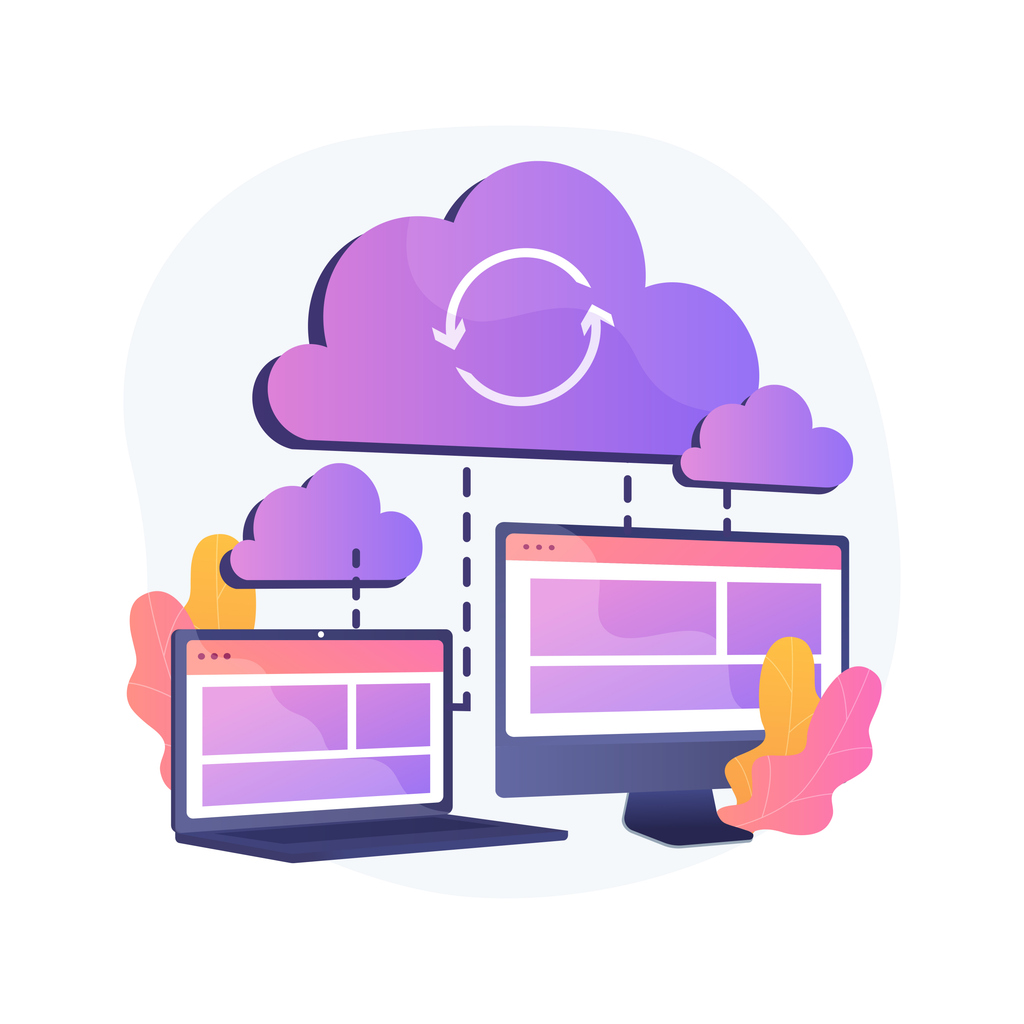
Remote work has become the norm, enabling teams to collaborate from anywhere in the world. However, managing software QA in a remote environment comes with its own set of challenges. From coordinating distributed teams to maintaining consistency in testing workflows, the shift to remote operations demands innovative solutions. Appvance IQ (AIQ), the industry’s leading AI-driven
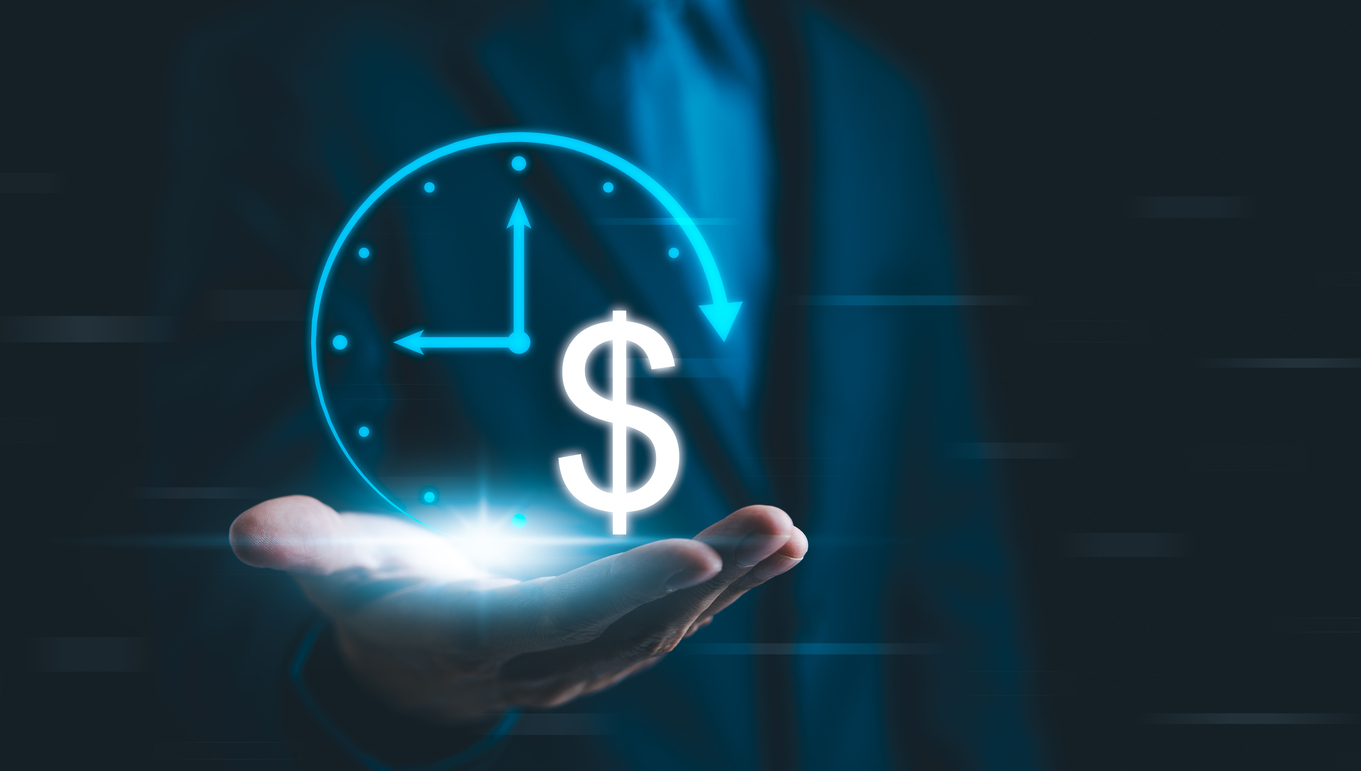
When it comes to software development, the ability to identify and address bugs quickly is paramount. Traditional exploratory testing, while effective, often requires significant time and resources. Appvance IQ (AIQ) revolutionizes this process with AI-powered exploratory testing, enabling faster bug discovery without human intervention and dramatically reducing time and costs. The Challenges of Traditional Exploratory
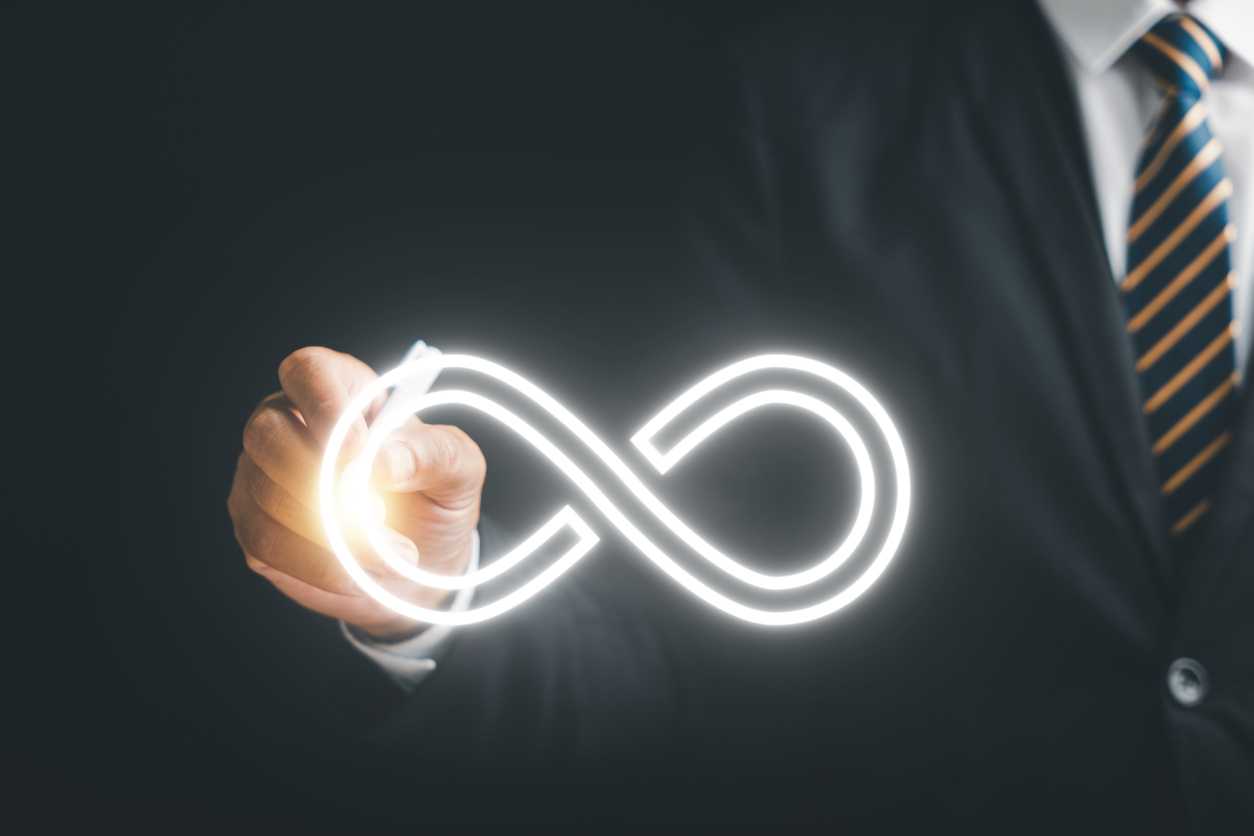
Continuous Integration (CI) is a cornerstone of modern software development, enabling teams to merge code changes frequently while maintaining a stable codebase. However, achieving true CI requires more than just frequent commits—it demands robust automated testing integrated seamlessly into your CI/CD pipeline. Enter Appvance IQ (AIQ), a game-changing platform that empowers businesses to achieve genuine
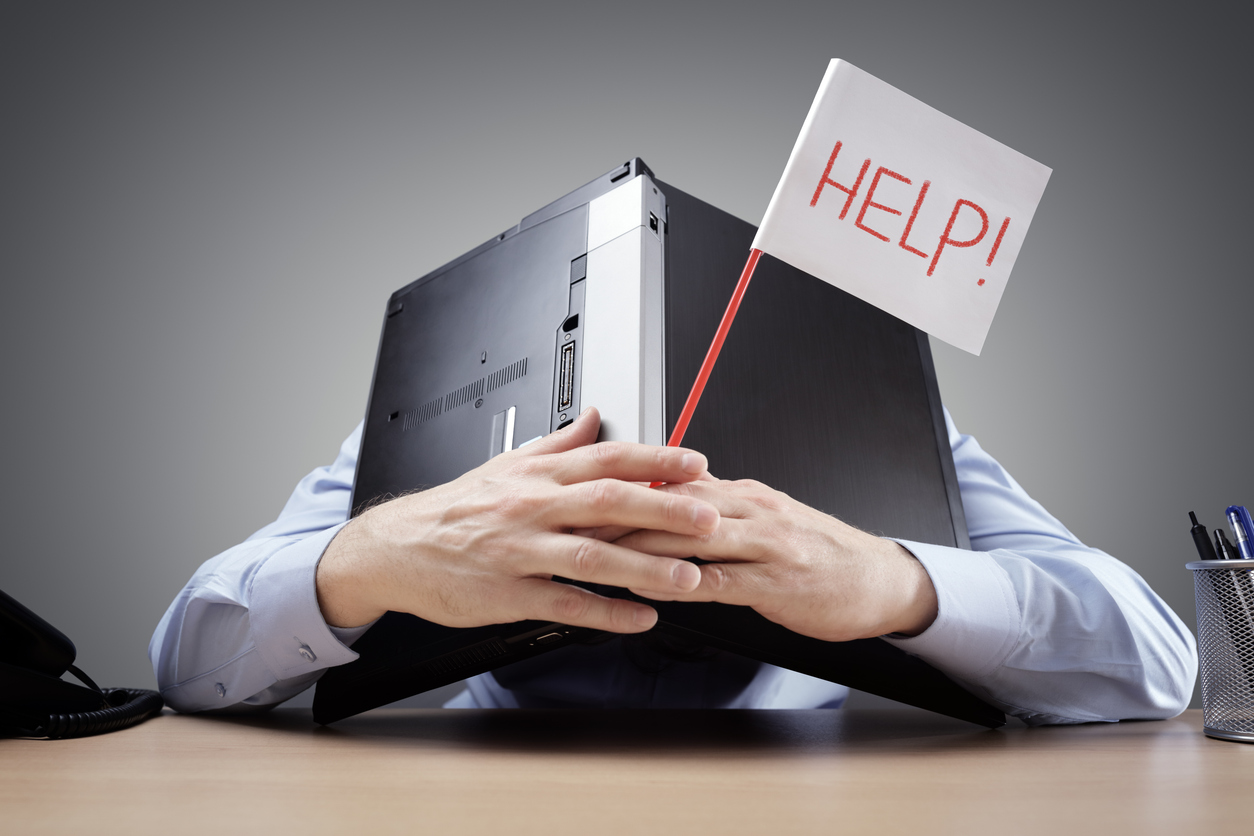
Technical debt is a term familiar to many development teams, referring to the long-term consequences of taking shortcuts in software development. While sometimes necessary to meet tight deadlines, this debt accumulates over time, leading to increased maintenance costs, reduced productivity, and greater risk of defects. Fortunately, the advent of AI-powered solutions like Appvance IQ (AIQ)
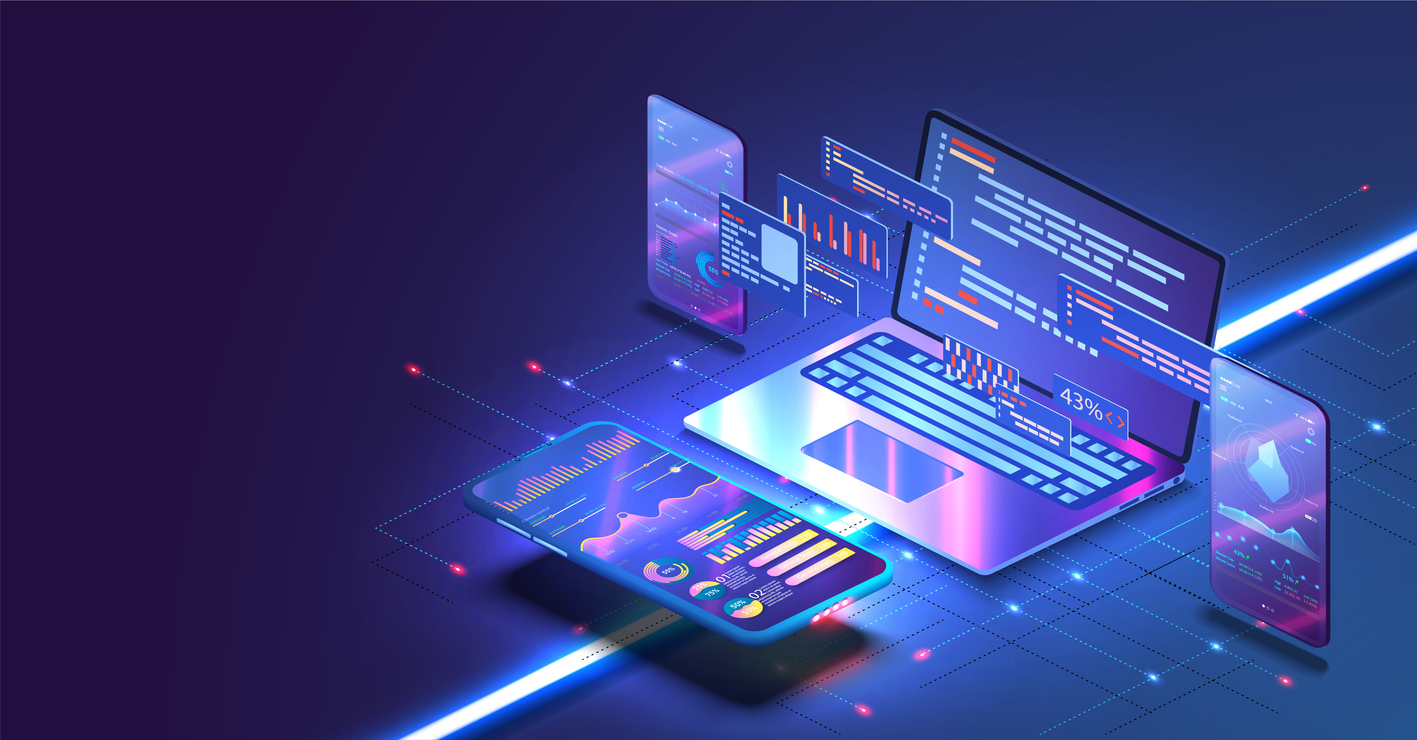
Enterprise applications are the backbone of modern businesses, supporting critical operations across diverse industries. However, their complexity and scale pose unique challenges for testing teams. Ensuring these applications perform seamlessly requires handling large volumes of test cases without sacrificing speed or performance. Appvance IQ (AIQ) is uniquely designed to scale automated testing to meet the
3080 Olcott Street
Suite B240
Santa Clara, CA 95054
[email protected] (855) 254-1164
3080 Olcott Street
Suite B240
Santa Clara, CA 95054
[email protected] (855) 254-1164
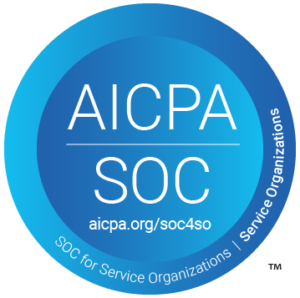
Product
- Autonomous Testing
- Test Creation
- Test Execution
- Test Results
Solutions
- By Technology
- By Use Cases
Resources
Company
Popular Links
- Autonomous Testing
- Test Creation
- Test Execution
- Test Results
© 2025 Appvance Inc. • All Rights Reserved.